早在2012的时候,iTunes 11就可以从封面中自动提取出主要的颜色用在歌曲列表里的字体和背景上了。
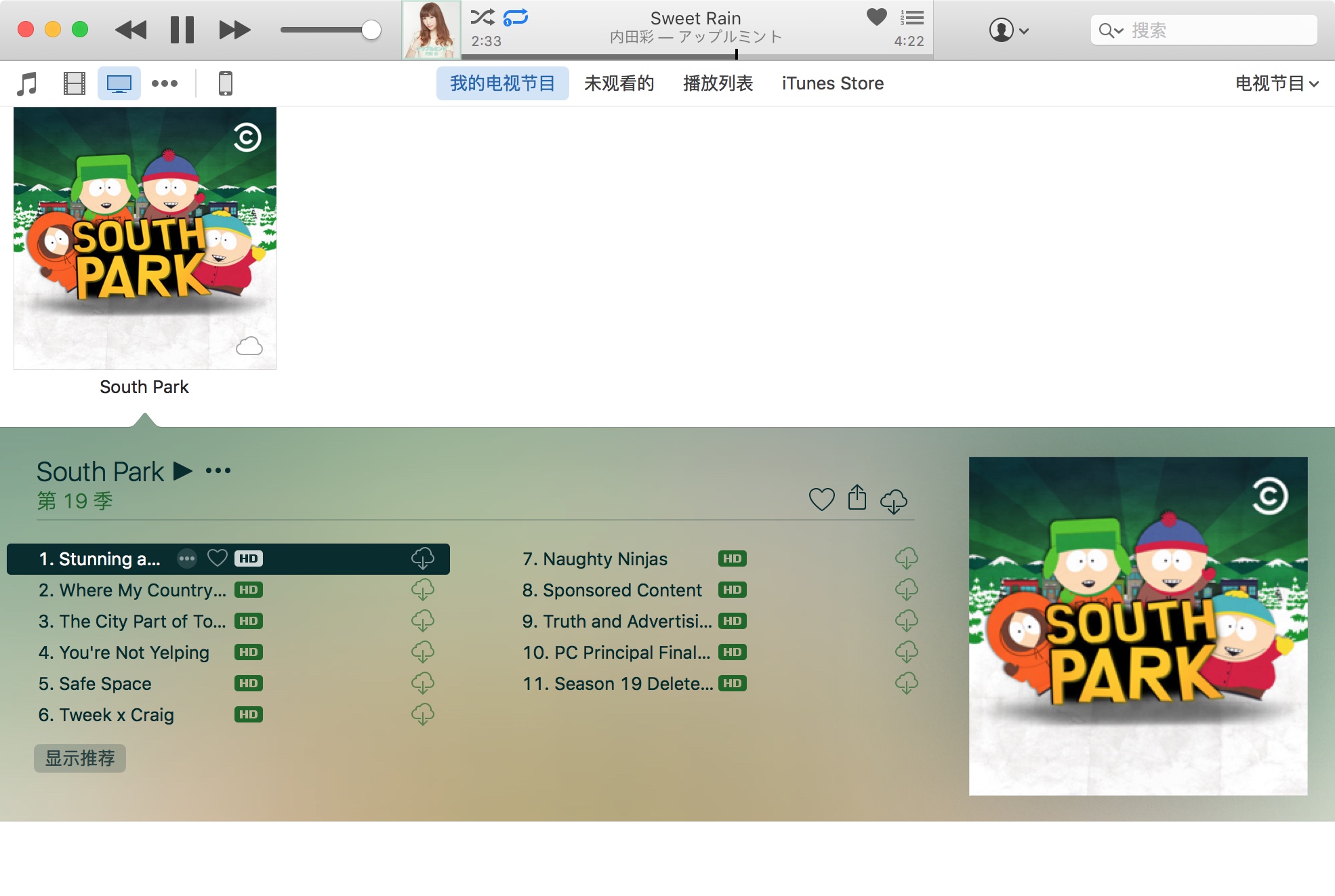
然而当时的我万万没有想到的是,如今自己也需要这样的算法了。
简单考虑了一下,打算用OpenCV读图,然后自己实现一下K-means算法来计算出给定图像中主要颜色。
对于聚类算法的综合性介绍可以参见这篇zhihu上的回答,用于数据挖掘的聚类算法有哪些,各有何优势?,及其这篇scikit-learn上的文章,scikit-learn Clustering¶。本文不再对聚类算法展开叙述。
其实整体思路很简单,先加载图片,然后按比例缩放为较小的图,随后统计小图中所有出现的颜色及其次数,从这些颜色中随机选k个作为初始条件开始进行K-means聚类,随机数用标准MersenneTwister PRNG,在计算距离时用L2 norm的欧式距离(Euclidean distance),最后以精度作为迭代结束的条件。
核心的K-means聚类算法就寥寥数十行,
Cluster mashiro::kmeans(const vector<MashiroColorWithCount>& pixels, std::uint32_t k, double min_diff) noexcept { Cluster clusters; uint32_t randmax = static_cast<uint32_t>(pixels.size()); // 使用标准MersenneTwister PRNG保证取的点的随机性 MersenneTwister mt(static_cast<uint32_t>(time(NULL))); // 取出k个点 for (uint32_t i = 0; i < k; i++) { auto iter = pixels.cbegin(); for (uint32_t t = 0; t < mt.rand() % randmax; t++, iter++); clusters.emplace_back(iter->first); } while (1) { ClusteredPoint points; // 与每一类的中心点比较距离, 找一个最邻近的类 for (auto iter = pixels.cbegin(); iter != pixels.cend(); iter++) { MashiroColor color = iter->first; double smallestDistance = DBL_MAX; double distance; uint32_t smallestIndex; for (uint32_t i = 0; i < k; i++) { distance = mashiro::euclidean(color, clusters[i]); if (distance < smallestDistance) { smallestDistance = distance; smallestIndex = i; } } points[smallestIndex].emplace_back(MashiroColorWithCount(color, iter->second)); } // 重新计算每类的中心值 double diff = 0; for (std::uint32_t i = 0; i < k; i++) { MashiroColor oldCenter = clusters[i]; MashiroColor newCenter = mashiro::center(points[i]); clusters[i] = newCenter; diff = max(diff, mashiro::euclidean(oldCenter, newCenter)); } // 当差距足够小时, 停止循环 if (diff < min_diff) { break; } } return clusters; }
你可以在我的 Git 上找到这个项目,mashiro
mashiro的API的形式也很简单
Mat image = imread("/PATH/TO/AN/IMAGE"); mashiro mashiro(image); mashiro.color(color, [](Mat& image, const Cluster colors){ for_each(colors.cbegin(), colors.cend(), [](const MashiroColor& color){ cout<<"(" <<color[0]<<", " <<color[1]<<", " <<color[2]<<")" <<endl; }); });
我们将用这张图来测试
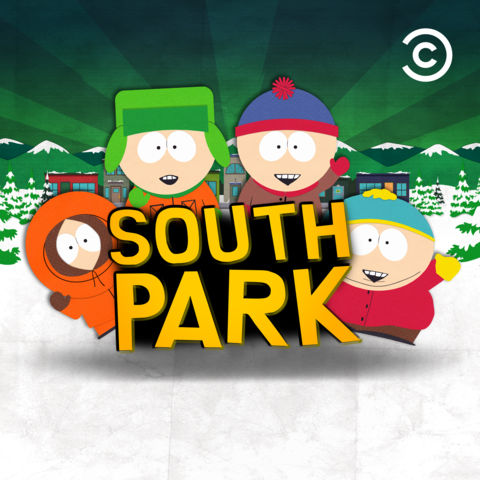
三个颜色中有两个比较像,不过都只是用在了很小的地方。
总的来说,表现还是可以,当然了,要想完全模仿iTunes那肯定是不可行的。
updated (2016-02-05):
mashiro在OS X下的Demo。
实际结果(图大小,3960x17475),这里为了直观的画出来,所以颜色只用了12种,类型是HSV,在分类时S, V都被设定为1,故黑色也被划到红色里去了。
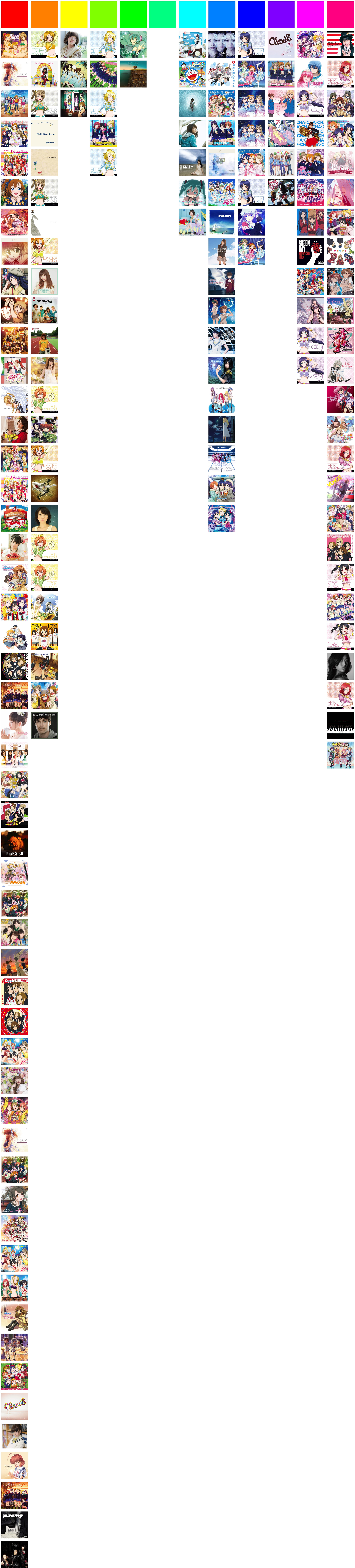
博主你好,在下载使用中发现,几个opencv库没有导入成功。是红色的需要做什么吗?